ALBA Synchrotron
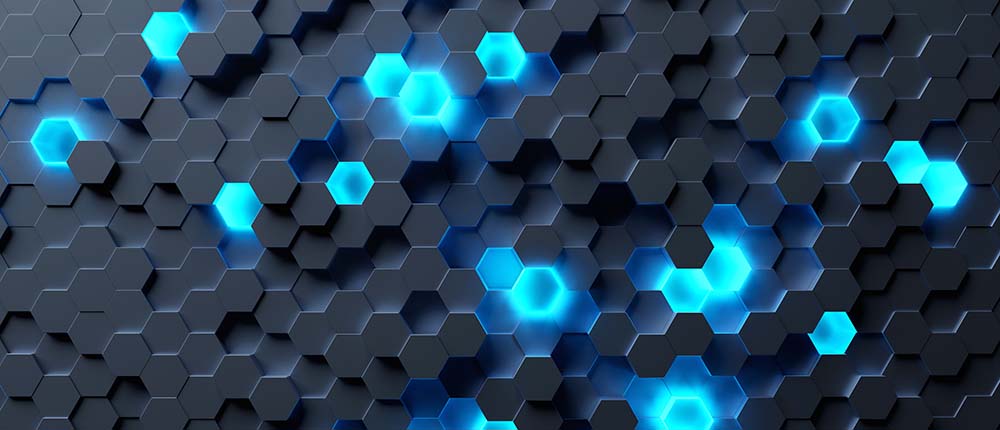
Sergio Santos (Department of Physics and Technology, UiT The Artic University of Norway, 9010, Tromso, Norway)
When
Location
Maxwell Auditorium
Where
ALBA Synchrotron
https://indico.cells.es/event/1651/
Infrastructure
- InCAEM project
Abstract
Multifrequency atomic force microscopy (AFM) generates multiple observables per pixel, capturing rich spectral and mechanical information beyond conventional single-channel imaging. While multifrequency AFM is traditionally employed for pixel-by-pixel quantification of material properties, its full potential extends beyond direct numerical extraction. We propose leveraging the inherent multifrequency data structure to first identify objects and distinguish physical phenomena before applying quantitative models.
By integrating AI vision techniques with machine learning algorithms, we process multiple observables per pixel to automatically detect features, classify material regions, identify physical transitions, and predict optimal force models in real time. This approach shifts the paradigm from direct parameter extraction to a two-step process: (1) object and phenomenon identification through vision-based, data-driven classification and (2) subsequent analytical quantification using the most suitable force model. We demonstrate that this method improves contrast, enhances the reliability of material property retrieval, and reveals nanoscale interactions that remain concealed in raw AFM channels.
The framework establishes an intelligent interpretation of multifrequency AFM data, enabling automated material classification and adaptive force modelling. It bridges the gap between high-resolution imaging and quantitative nanomechanical analysis, paving the way for autonomous, AI-enhanced AFM-based characterization techniques.
- Santos, S.; Elsherbiny, L.; Lai, C.-Y.; Askar, K.; Gadelrab, K.; Chiesa, M., Automatic Generation of Contrast Maps in Terms of Van Der Waals Material Properties in Bimodal Afm. The Journal of Physical Chemistry C 2024, 128, 21154-21163
- Elsherbiny, L.; Santos, S.; Gadelrab, K.; Olukan, T.; Font, J.; Barcons, V.; Chiesa, M., Machine Learning Assisted Multifrequency Afm: Force Model Prediction. Applied Physics Letters 2023, 123.
- Lai, C.-Y, Santos, S.; Elsherbiny, L.; Gadelrab, K.; Chiesa, M., Intelligent Nanoscale Imaging: Harnessing Machine Learning for Multifrequency AFM (2025) Under preparation.